About
RADAREYE is a startup focused on commercialising recent breakthroughs in cross-modal radar-camera self-supervised learning.
The company is targeting the automotive market as an immediate route to the commercialisation of its technology, with medium-term plans for home automation, healthcare, smart cities, and industrial surveillance applications.
Product
Technology
The Radium approach
Radar is not susceptible to the same limitations as cameras — it can see through bad weather like fog and detect cars.
We have built an ML model that is able take the radar data and recognise the patterns that represent cars.
The output of our model is the position of cars. This inference is used within car navigation system.
Radium in a nutshell
Figures show how we train Radium (on the left), and how we deploy Radium (on the right). Foggy images are the reference visual scenes of the radar heatmaps on the right. Radar allows us to accurately detect and localise cars under bad visibility, whereas detection is not viable from the corresponding foggy images.
Team
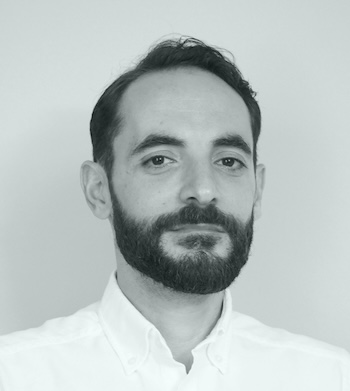
Mo Alloulah
Founder & CEO
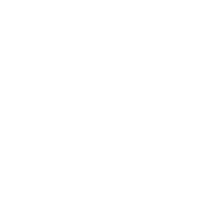
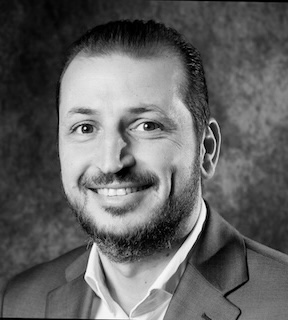
Rani Mouchamel
Co-Founder & COO
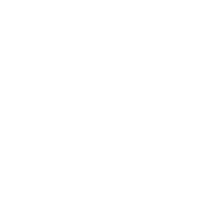
RADAREYE’s co-founders have 44+ years combined experience in silicon, radar, perception, automotive, operations, and leardership, most recently at Nokia Bell Labs and General Motors. The company is based in London.
Advisors
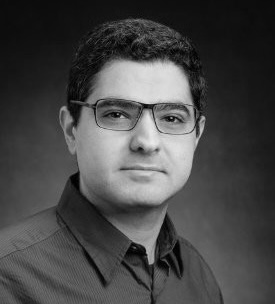
Haitham Hassanieh
Professor, EPFL
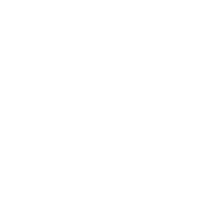
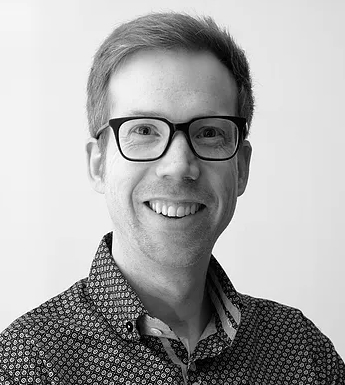
Dominique Guinard
VP Innovation, Digimarc
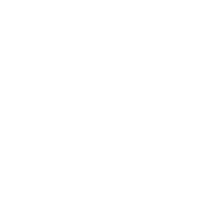
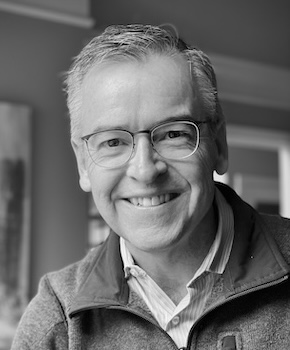
Colm Boran
Chief Engineer (ret.)
Autonomous/ADAS
Ford Motor Company
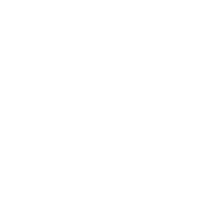
RADAREYE is fortunate to be advised by a top academic, a London-based startup co-founder with successful exit, and a veteran of the automotive industry.
Trusted Partners
Publications
info@radareye.ai
Elements
Text
This is bold and this is strong. This is italic and this is emphasized. This is superscript text and this is subscript text. This is underlined and this is code: for (;;) { ... }
. Finally, this is a link.
Heading Level 2
Heading Level 3
Heading Level 4
Heading Level 5
Heading Level 6
Blockquote
Fringilla nisl. Donec accumsan interdum nisi, quis tincidunt felis sagittis eget tempus euismod. Vestibulum ante ipsum primis in faucibus vestibulum. Blandit adipiscing eu felis iaculis volutpat ac adipiscing accumsan faucibus. Vestibulum ante ipsum primis in faucibus lorem ipsum dolor sit amet nullam adipiscing eu felis.
Preformatted
i = 0;
while (!deck.isInOrder()) {
print 'Iteration ' + i;
deck.shuffle();
i++;
}
print 'It took ' + i + ' iterations to sort the deck.';
Lists
Unordered
- Dolor pulvinar etiam.
- Sagittis adipiscing.
- Felis enim feugiat.
Alternate
- Dolor pulvinar etiam.
- Sagittis adipiscing.
- Felis enim feugiat.
Ordered
- Dolor pulvinar etiam.
- Etiam vel felis viverra.
- Felis enim feugiat.
- Dolor pulvinar etiam.
- Etiam vel felis lorem.
- Felis enim et feugiat.
Icons
Actions
Table
Default
Name |
Description |
Price |
Item One |
Ante turpis integer aliquet porttitor. |
29.99 |
Item Two |
Vis ac commodo adipiscing arcu aliquet. |
19.99 |
Item Three |
Morbi faucibus arcu accumsan lorem. |
29.99 |
Item Four |
Vitae integer tempus condimentum. |
19.99 |
Item Five |
Ante turpis integer aliquet porttitor. |
29.99 |
|
100.00 |
Alternate
Name |
Description |
Price |
Item One |
Ante turpis integer aliquet porttitor. |
29.99 |
Item Two |
Vis ac commodo adipiscing arcu aliquet. |
19.99 |
Item Three |
Morbi faucibus arcu accumsan lorem. |
29.99 |
Item Four |
Vitae integer tempus condimentum. |
19.99 |
Item Five |
Ante turpis integer aliquet porttitor. |
29.99 |
|
100.00 |